- Home
- Search
- Quanquan Gu
- All Reviews
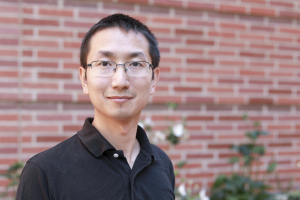
Quanquan Gu
AD
Based on 20 Users
The professor did a decent job explaining the concepts of conventional AI and showing the applications of these algorithms. The first part of this class is taught with lisp, an oldish programming language, which could be replaced by some modern languages. The second part is more about logic and the professor is excellent at extending this to modern AI tasks. There are attendance quizzes helping us review. Midterm and final are easy, and the professor is helpful making accommodations. Discussion should be better to host in person, but TAs are nice explaining the requirements of the homework.
Professor is not a great lecturer and the slides/lectures are pretty boring. Somehow despite being surface-level info and overviews, they are still too "in it" to be interesting. In depth examples appear on slides in place of actually helpful overall rules.
That being said, the class is still pretty easy if you read the textbook, google the terms that come up in the assignments, and browse the slides. I didn't even watch most of the lectures.
I found this class very interesting, although the content of the course covered a lot of history and more antiquated AI algorithms. However, this does not mean the material will always be like this in the future, and I still think it was a good experience regardless. The projects were interesting, homework was manageable, and the exams were very fair (I do recommend making a study guide!!!). Also, LISP was used, so I guess beware of that. My experience was also made better because my TA for the class was excellent, so I would say going to discussion is worth it, but YMMV.
Lectures: He is not the best lecturer. For the more mathematical/theoretical content, I learned more from the book. For the content on neural networks, he didn’t even seem familiar with the slides. But the networks content also is only on quizzes, so I guess it’s ok.
Homework 35%: They are very mathematical questions until the switch into talking about networks, then the homework becomes implementing networks by scratch in Python. It was also a grind for the math homeworks because they were required to be in LaTeX.
Midterm 30%: They generously gave a very similar practice midterm, so as long as you can confidently solve those problems, or at least note how to solve them on your cheatsheet, you’ll score well. It also covers the math content more.
No final, 6 quizzes that are only worth 5% total of grade.
Final project 30%: You can choose from some given proposals that Prof. asked people he knows to provide some ideas for, or pursue your own project if you’re smart. Would recommend trying to take the course with friends/colleagues you trust, so that you can collaborate with no issues.
Overall, I would recommend this class if you are mathematically mature, have an interest in the math in ML theory, or are just very ML-invested. Or have an interest in self-learning all the math and network concepts. I unfortunately have little qualification in any of the above, so I suffered silently. Sunk cost fallacy is real. Shoutout to Lucas Tecot for the HW hints.
First time teaching so it was a easy class. Gu goes pretty slow and isn’t the most engaging lecturer. Most people chose not to go to class and just put everything on the cheat sheet. Exam questions come straight from his PowerPoint so if you can copy all the information it’s pretty easy to ace. Projects can all be found on GitHub.
I stopped going to class around Week 2, because it's just impossible to stay awake with his teaching. Most topics can be learned from the slides, but I struggled with reasoning under uncertainty and after. Homeworks were from past quarters. Midterm was also derived from past exams and is pretty simple if you're comfortable with tracing through searches, backtracking, alpha-beta pruning, and putting the rest of conceptual info on a cheat sheet. Lisp is absolute ass and I hated HW 2 and 3. The conceptual HWs on logic, 5 and 6, also sucked because you had to provide way too much work for your answers. In conclusion, take this class with Gu if you've become accustomed to learning content on your own and then suffering through homeworks alone. Shoutout to corona for saving me from taking the final.
I absolutely loved this class and felt that I learned a lot from it. I was really excited about the topics covered in this course, like constraint-satisfaction problems, all the different types of search algorithms, first-order logic, and Bayesian nets. This course really teaches you many basic and useful techniques in classical AI.
Professor Gu is truly amazing. He made the lectures interesting and gave a lot of good insights and examples on the topics. During the lecture, he always took time to slow down and made sure that all questions were answered. He also gave extra office hours when the material got harder. He is very helpful, intelligent, and truly cares about his students.
The professor did a decent job explaining the concepts of conventional AI and showing the applications of these algorithms. The first part of this class is taught with lisp, an oldish programming language, which could be replaced by some modern languages. The second part is more about logic and the professor is excellent at extending this to modern AI tasks. There are attendance quizzes helping us review. Midterm and final are easy, and the professor is helpful making accommodations. Discussion should be better to host in person, but TAs are nice explaining the requirements of the homework.
Professor is not a great lecturer and the slides/lectures are pretty boring. Somehow despite being surface-level info and overviews, they are still too "in it" to be interesting. In depth examples appear on slides in place of actually helpful overall rules.
That being said, the class is still pretty easy if you read the textbook, google the terms that come up in the assignments, and browse the slides. I didn't even watch most of the lectures.
I found this class very interesting, although the content of the course covered a lot of history and more antiquated AI algorithms. However, this does not mean the material will always be like this in the future, and I still think it was a good experience regardless. The projects were interesting, homework was manageable, and the exams were very fair (I do recommend making a study guide!!!). Also, LISP was used, so I guess beware of that. My experience was also made better because my TA for the class was excellent, so I would say going to discussion is worth it, but YMMV.
Lectures: He is not the best lecturer. For the more mathematical/theoretical content, I learned more from the book. For the content on neural networks, he didn’t even seem familiar with the slides. But the networks content also is only on quizzes, so I guess it’s ok.
Homework 35%: They are very mathematical questions until the switch into talking about networks, then the homework becomes implementing networks by scratch in Python. It was also a grind for the math homeworks because they were required to be in LaTeX.
Midterm 30%: They generously gave a very similar practice midterm, so as long as you can confidently solve those problems, or at least note how to solve them on your cheatsheet, you’ll score well. It also covers the math content more.
No final, 6 quizzes that are only worth 5% total of grade.
Final project 30%: You can choose from some given proposals that Prof. asked people he knows to provide some ideas for, or pursue your own project if you’re smart. Would recommend trying to take the course with friends/colleagues you trust, so that you can collaborate with no issues.
Overall, I would recommend this class if you are mathematically mature, have an interest in the math in ML theory, or are just very ML-invested. Or have an interest in self-learning all the math and network concepts. I unfortunately have little qualification in any of the above, so I suffered silently. Sunk cost fallacy is real. Shoutout to Lucas Tecot for the HW hints.
First time teaching so it was a easy class. Gu goes pretty slow and isn’t the most engaging lecturer. Most people chose not to go to class and just put everything on the cheat sheet. Exam questions come straight from his PowerPoint so if you can copy all the information it’s pretty easy to ace. Projects can all be found on GitHub.
I stopped going to class around Week 2, because it's just impossible to stay awake with his teaching. Most topics can be learned from the slides, but I struggled with reasoning under uncertainty and after. Homeworks were from past quarters. Midterm was also derived from past exams and is pretty simple if you're comfortable with tracing through searches, backtracking, alpha-beta pruning, and putting the rest of conceptual info on a cheat sheet. Lisp is absolute ass and I hated HW 2 and 3. The conceptual HWs on logic, 5 and 6, also sucked because you had to provide way too much work for your answers. In conclusion, take this class with Gu if you've become accustomed to learning content on your own and then suffering through homeworks alone. Shoutout to corona for saving me from taking the final.
I absolutely loved this class and felt that I learned a lot from it. I was really excited about the topics covered in this course, like constraint-satisfaction problems, all the different types of search algorithms, first-order logic, and Bayesian nets. This course really teaches you many basic and useful techniques in classical AI.
Professor Gu is truly amazing. He made the lectures interesting and gave a lot of good insights and examples on the topics. During the lecture, he always took time to slow down and made sure that all questions were answered. He also gave extra office hours when the material got harder. He is very helpful, intelligent, and truly cares about his students.