- Home
- Search
- Jonathan C Kao
- All Reviews
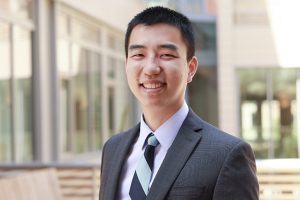
Jonathan Kao
AD
Based on 89 Users
Be prepared to spend 20+ hours a week on the homework assignments. I learned a ton from this course. It makes it to where AI/ML is not a black box anymore. You can understand how things are working and how it all comes back to the math.
The lectures are very good. The professor and TAs are very helpful. It is a great course which I would recommend if you are single and have the time.
I would highly recommend this class to any interested in deep learning and machine learning. Professor Kao is a very good lecturer and he does an amazing job explaining concepts. I never truly understood how backpropagation worked until he explained it in class. Anyone interested in research/ML should definitely take this class. You will learn so much.
However, the class is not a cake walk. It's actually fairly easy to get a good grade in this class as long as you put in the effort. There is only one exam around week 8, which won't be bad if you pay attention to lecture (our average for the exam was a 95%). The homeworks are the real killer and can take a very long time. You essentially have to build neural networks from scratch using Python and Numpy.
Overall, this is an amazing class where you can truly learn so much, but at the price of many hours of homework. Professor Kao is probably one of my favorite professors I have ever had at UCLA.
I think the winter 2024 offering of C147 was very similar to the reviews I've read in the past:
- There were some very hard/long assignments. However if you used Piazza you should be fine
- The class was a bit more mathy in the beginning than compared to the end
- The professor was excellent!
There are also several comments complaining about the "slow pace" of the class, "over-enrollment" and new 50% Midterm Weight. I'd like to address those and what my views are as a fellow student:
Personally, as someone who skipped M146 and went straight into C147, I appreciated that the professor reviewed some of the more fundamental aspects of DL in the beginning of the course. Furthermore, I'd like to point out that this is after all, a graduate class. Grad Students often don't get to take the particular sequence of courses at UCLA prior to coming here, so it make sense that the professor do some review in the very beginning to make sure everyone is on the same page.
For those that were interested in "practical" machine learning (ie learning one of the popular ML libs), the final project was an opportunity to do that. Personally, I think this class is not a torch-bootcamp and rightfully so: C147 focus on more fundamental knowledge and the theory side of DL. There are also other more coding-heavy classes offered (CS188 in W24 for example) that one can take to gain that kind of experience.
While it is true this was a very big class, personally between taking the class vs not taking the class I'd always choose the former. I definitely appreciated the effort the teaching staff put into making the logistics work for such a large class!
Finally, the median for the class was an A- (partly due to grad class, also because Kao doesn't grade harsh). I really don't understand why people complain about grades at this point considering this is an upper-div and the midterm median was a B.
I don't have anything to say that others haven't already said, Professor Kao is truly one of the best lecturers at UCLA and I would highly recommend this class if you are interested in Neural Networks and Deep Learning. Also, the TAs for this class were amazing, especially Tonmoy Monsoor. Tonmoy is insanely knowledgeable about the topic and his discussions were super useful for the homeworks!
Grading:
Homework: 40% (5 homeworks)
Midterm: 30%
Final Project: 30%
Extra Credit: 0.5% for filling out class eval, up to 1.5% for participating on piazza (in a useful way), and some extra credit given on the midterm (final question on the exam is optional extra credit)
Jonathan Kao is my favorite professor at UCLA. Highly recommend this organized, fair, and passionate lecturer.
The professor and TA's are AMAZING, and I learned a lot from this class. However, words cannot explain how severely I disagree with the syllabus and logistics of this course. I think it was a bad idea to have a midterm be worth 50% of your grade. I understand that they changed it from 30% to 50% to even out the distribution or whatever, but it doesn't really make sense to up it by 20%. I feel like 40% would have been a lot more fair. Other than that glaring issue, this course is great and Prof Kao is extremely helpful and kind and approachable. I'd recommend this course to anyone interested in ML.
I imagine this class was much better in the past, but this iteration was just awful. Unenforced prerequisites led to a massive class (over 400!) of folks who weren't familiar with linear algebra/matrix calculus asking questions about course prerequisites NONSTOP, and Prof Kao having to explain these. We ended up falling behind by 8 lecture hours (4 lectures of 2 hours) compared to last year's iteration because of this.
Additionally, it seems Prof Kao experimented with asking more open ended exam questions this year compared to the last few. This is totally fine, except he added a closed solution rubric?? Exam average this time was low 80s (worth 50% of the grade => the grade distribution will be MUCH lower this time), and led to Piazza being spammed endlessly for students seeking additional 1.5% extra credit (no, I don't want nor need an Excel spreadsheet to calculate my course grade; stop posting this on a Q&A board). This is not how you run a class, this was a mess and easily one of the biggest wastes of time for anyone who has taken M146.
If the department doesn't enforce prerequisites nor cap the class size next year, DO NOT TAKE THIS.
Like everyone said, Kao is probably one of the nicest professors I've ever met. While some professors care only about research, it's clear that Kao genuinely cares about teaching. He wants us to learn in an intellectually honest way and grasp the material conceptually. He encourages everyone to come to office hours, he encourages people to ask questions, and he is so super approachable. He has a forgiving late day policy and will give class wide extensions if the class expresses a need. He wants everyone in the class to succeed. The material is challenging but he makes it super accessible and it's super interesting.
The class is 40% homework which sounds nice but I found the homeworks to be harder than the tests because they were long and they had us work on content we literally just learned. You MUST start early. I would say to start immediately, as homeworks are usually assigned Thursdays, do as much as you can over the weekend, and then go to the TA's or his office hours during the week to answer questions. It is very possible that you won't be able to do a lot of the homework alone/when you first look at it, but he and the TAs are VERY helpful and will guide you to the right answer. I like to think I'm not an idiot, and I absolutely could not have done these homeworks without going to office hours.
The rest of the class is 25% midterm and 35% final. After the midterm, he said that if we performed better on the final, he would replace our midterm score with our final score (he's super supportive and on the student's side!). As more Fall 2023 reviews come in, I expect people to say the midterm was easy and the final was hard. I don't know that I will completely agree. The midterm was extremely straightforward, as the TAs held a review session before the midterm that had problems that were almost identical to the real midterm. Thus, many people left after 40 minutes, and the median on the midterm was a 92. I think Kao reacted appropriately and adjusted the difficulty of the final to make it more challenging. I also think a lot of people were expecting the final to be just as easy and it was not. The content on the final is just in general more challenging, but we also weren't spoon fed the entire final like we were the midterm. Thus, many people freaked out after the final and said it was super hard, and I don't think this is super accurate. The final did have a much lower average than the midterm (in the 70s I believe) but I just feel like a 92 median is high in general. There was not a single question on the final that was completely out of left field -- I genuinely believe every question was fair game. Thus, main point is: go to office hours, go to TA review sessions, do EVERY SINGLE practice midterm/final/discussion/homework. And make sure you KNOW the material from a CONCEPTUAL STANDPOINT. To study for the final, I went through every single discussion worksheet, homework, and the past 4 years of finals (he provides them god BLESS) and made sure I understood everything. Also, on your cheat sheet, do NOT just put formulas. The tests test HOW YOU APPLY THE CONCEPTS. He will NOT just be like "State the convolution theorem :D (10pts)." So look at the midterm and final answer keys copy solutions of different types of problems, it will really help you out. Good luck, and really take advantage of what an amazing teacher he is!
The other reviews pretty much sum him up well. This class is really, really hard, but I don’t think anyone else in the department can teach it better than him. So, I’d highly reccommend
I love Jonathan Kao. His lectures are very clear—with amazing annotated notes. Concepts that may seem confusing—Kao has a unique ability to make them seem approachable and common sense-like.
In office hours, he is always willing to take questions, talk about the course, or just life in general. I've had great conversations with him regarding the existence of free will. Not many professors are that engaged with their students.
One minor criticism I have of Kao is how he takes questions in lecture. He indulges in almost every single question, which slows down lecture tremendously. It's great he wants to resolve any unanswered questions, but it's just too many. (It's also evident that some students ask questions just to make them seem smarter to the professor, but that's another concern.)
I feel like the professor can fix this by setting expectations for questions at the beginning of the course. If you feel like your question helps everyone in class, feel free to ask it in lecture. If not, ask it during office hours.
Overall though, great professor.
I love Tonmoy Monsoor. Super knowledgeable TA, always willing to help during discussion, holds great review sessions.
Be prepared to spend 20+ hours a week on the homework assignments. I learned a ton from this course. It makes it to where AI/ML is not a black box anymore. You can understand how things are working and how it all comes back to the math.
The lectures are very good. The professor and TAs are very helpful. It is a great course which I would recommend if you are single and have the time.
I would highly recommend this class to any interested in deep learning and machine learning. Professor Kao is a very good lecturer and he does an amazing job explaining concepts. I never truly understood how backpropagation worked until he explained it in class. Anyone interested in research/ML should definitely take this class. You will learn so much.
However, the class is not a cake walk. It's actually fairly easy to get a good grade in this class as long as you put in the effort. There is only one exam around week 8, which won't be bad if you pay attention to lecture (our average for the exam was a 95%). The homeworks are the real killer and can take a very long time. You essentially have to build neural networks from scratch using Python and Numpy.
Overall, this is an amazing class where you can truly learn so much, but at the price of many hours of homework. Professor Kao is probably one of my favorite professors I have ever had at UCLA.
I think the winter 2024 offering of C147 was very similar to the reviews I've read in the past:
- There were some very hard/long assignments. However if you used Piazza you should be fine
- The class was a bit more mathy in the beginning than compared to the end
- The professor was excellent!
There are also several comments complaining about the "slow pace" of the class, "over-enrollment" and new 50% Midterm Weight. I'd like to address those and what my views are as a fellow student:
Personally, as someone who skipped M146 and went straight into C147, I appreciated that the professor reviewed some of the more fundamental aspects of DL in the beginning of the course. Furthermore, I'd like to point out that this is after all, a graduate class. Grad Students often don't get to take the particular sequence of courses at UCLA prior to coming here, so it make sense that the professor do some review in the very beginning to make sure everyone is on the same page.
For those that were interested in "practical" machine learning (ie learning one of the popular ML libs), the final project was an opportunity to do that. Personally, I think this class is not a torch-bootcamp and rightfully so: C147 focus on more fundamental knowledge and the theory side of DL. There are also other more coding-heavy classes offered (CS188 in W24 for example) that one can take to gain that kind of experience.
While it is true this was a very big class, personally between taking the class vs not taking the class I'd always choose the former. I definitely appreciated the effort the teaching staff put into making the logistics work for such a large class!
Finally, the median for the class was an A- (partly due to grad class, also because Kao doesn't grade harsh). I really don't understand why people complain about grades at this point considering this is an upper-div and the midterm median was a B.
I don't have anything to say that others haven't already said, Professor Kao is truly one of the best lecturers at UCLA and I would highly recommend this class if you are interested in Neural Networks and Deep Learning. Also, the TAs for this class were amazing, especially Tonmoy Monsoor. Tonmoy is insanely knowledgeable about the topic and his discussions were super useful for the homeworks!
Grading:
Homework: 40% (5 homeworks)
Midterm: 30%
Final Project: 30%
Extra Credit: 0.5% for filling out class eval, up to 1.5% for participating on piazza (in a useful way), and some extra credit given on the midterm (final question on the exam is optional extra credit)
The professor and TA's are AMAZING, and I learned a lot from this class. However, words cannot explain how severely I disagree with the syllabus and logistics of this course. I think it was a bad idea to have a midterm be worth 50% of your grade. I understand that they changed it from 30% to 50% to even out the distribution or whatever, but it doesn't really make sense to up it by 20%. I feel like 40% would have been a lot more fair. Other than that glaring issue, this course is great and Prof Kao is extremely helpful and kind and approachable. I'd recommend this course to anyone interested in ML.
I imagine this class was much better in the past, but this iteration was just awful. Unenforced prerequisites led to a massive class (over 400!) of folks who weren't familiar with linear algebra/matrix calculus asking questions about course prerequisites NONSTOP, and Prof Kao having to explain these. We ended up falling behind by 8 lecture hours (4 lectures of 2 hours) compared to last year's iteration because of this.
Additionally, it seems Prof Kao experimented with asking more open ended exam questions this year compared to the last few. This is totally fine, except he added a closed solution rubric?? Exam average this time was low 80s (worth 50% of the grade => the grade distribution will be MUCH lower this time), and led to Piazza being spammed endlessly for students seeking additional 1.5% extra credit (no, I don't want nor need an Excel spreadsheet to calculate my course grade; stop posting this on a Q&A board). This is not how you run a class, this was a mess and easily one of the biggest wastes of time for anyone who has taken M146.
If the department doesn't enforce prerequisites nor cap the class size next year, DO NOT TAKE THIS.
Like everyone said, Kao is probably one of the nicest professors I've ever met. While some professors care only about research, it's clear that Kao genuinely cares about teaching. He wants us to learn in an intellectually honest way and grasp the material conceptually. He encourages everyone to come to office hours, he encourages people to ask questions, and he is so super approachable. He has a forgiving late day policy and will give class wide extensions if the class expresses a need. He wants everyone in the class to succeed. The material is challenging but he makes it super accessible and it's super interesting.
The class is 40% homework which sounds nice but I found the homeworks to be harder than the tests because they were long and they had us work on content we literally just learned. You MUST start early. I would say to start immediately, as homeworks are usually assigned Thursdays, do as much as you can over the weekend, and then go to the TA's or his office hours during the week to answer questions. It is very possible that you won't be able to do a lot of the homework alone/when you first look at it, but he and the TAs are VERY helpful and will guide you to the right answer. I like to think I'm not an idiot, and I absolutely could not have done these homeworks without going to office hours.
The rest of the class is 25% midterm and 35% final. After the midterm, he said that if we performed better on the final, he would replace our midterm score with our final score (he's super supportive and on the student's side!). As more Fall 2023 reviews come in, I expect people to say the midterm was easy and the final was hard. I don't know that I will completely agree. The midterm was extremely straightforward, as the TAs held a review session before the midterm that had problems that were almost identical to the real midterm. Thus, many people left after 40 minutes, and the median on the midterm was a 92. I think Kao reacted appropriately and adjusted the difficulty of the final to make it more challenging. I also think a lot of people were expecting the final to be just as easy and it was not. The content on the final is just in general more challenging, but we also weren't spoon fed the entire final like we were the midterm. Thus, many people freaked out after the final and said it was super hard, and I don't think this is super accurate. The final did have a much lower average than the midterm (in the 70s I believe) but I just feel like a 92 median is high in general. There was not a single question on the final that was completely out of left field -- I genuinely believe every question was fair game. Thus, main point is: go to office hours, go to TA review sessions, do EVERY SINGLE practice midterm/final/discussion/homework. And make sure you KNOW the material from a CONCEPTUAL STANDPOINT. To study for the final, I went through every single discussion worksheet, homework, and the past 4 years of finals (he provides them god BLESS) and made sure I understood everything. Also, on your cheat sheet, do NOT just put formulas. The tests test HOW YOU APPLY THE CONCEPTS. He will NOT just be like "State the convolution theorem :D (10pts)." So look at the midterm and final answer keys copy solutions of different types of problems, it will really help you out. Good luck, and really take advantage of what an amazing teacher he is!
I love Jonathan Kao. His lectures are very clear—with amazing annotated notes. Concepts that may seem confusing—Kao has a unique ability to make them seem approachable and common sense-like.
In office hours, he is always willing to take questions, talk about the course, or just life in general. I've had great conversations with him regarding the existence of free will. Not many professors are that engaged with their students.
One minor criticism I have of Kao is how he takes questions in lecture. He indulges in almost every single question, which slows down lecture tremendously. It's great he wants to resolve any unanswered questions, but it's just too many. (It's also evident that some students ask questions just to make them seem smarter to the professor, but that's another concern.)
I feel like the professor can fix this by setting expectations for questions at the beginning of the course. If you feel like your question helps everyone in class, feel free to ask it in lecture. If not, ask it during office hours.
Overall though, great professor.
I love Tonmoy Monsoor. Super knowledgeable TA, always willing to help during discussion, holds great review sessions.